A Game-Changing Discovery by Evan Miller
In the realm of artificial intelligence (AI), every detail counts. A tiny change can have a significant impact, and that’s exactly what Evan Miller, a renowned AI researcher, recently put forward in his attention-grabbing article, “Attention Is Off By One“. In it, Miller makes a bold claim about a minor modification that could transform how AI models operate, potentially leading to significant advancements in the field.
To understand Miller’s claim, let’s first understand what’s at the center of it: a component in AI called the “attention mechanism”. This mechanism allows an AI model to focus on the most important bits of data it’s working with. Imagine being at a busy party with multiple conversations happening at once. Your brain naturally filters out the background noise, allowing you to focus on the conversation you’re part of. The attention mechanism does something similar for AI.
There’s a specific type of AI model, called a Transformer, that uses this attention mechanism. Transformers are a bit like social butterflies at that busy party—they can participate in multiple conversations at the same time.
However, according to Miller, there’s a bug in the system. When a Transformer uses the attention mechanism, it’s essentially making a decision about what data is important and what’s not. But, just like a teacher who insists every student must answer every question, the current system forces each part of the Transformer to always contribute information. This often leads to the AI producing some extreme values that are much larger than their peers, somewhat akin to a student yelling out wildly incorrect answers.
Miller’s proposed solution to this problem is surprisingly simple: a minor tweak to the decision-making function of the AI, called the “softmax function”. He proposes adding a “+1” in the denominator of the softmax function. This seemingly minor change has the potential to allow parts of the Transformer to choose not to contribute information when it’s better not to, just as a student might choose not to answer a question they know nothing about.
Miller has named this new function “softmax1”. He believes that it could help resolve the issue with the extreme values in the models, making the AI more accurate and efficient.
A Potential Solution to an Overlooked Problem
The problem Miller identifies, while technical in nature, has significant real-world implications. AI models, particularly those using attention mechanisms like Transformer models, are being used in an increasing range of applications, from virtual assistants and language translation services to data analysis and decision-making tools.
The presence of extreme values or “outliers” in the output of these models can affect their performance and accuracy. This is similar to having a few overly dominant voices in a group discussion, which can skew the conversation and lead to less balanced and representative outcomes.
Until now, this issue has been somewhat overlooked. Miller’s proposed solution, the softmax1 function, represents a novel approach to this problem. If his theory is correct, it could help to balance the output of these AI models, just as ensuring that all voices are heard can lead to a more balanced discussion in a group.
Towards a More Efficient AI Future
Miller’s findings also have potential implications for the efficiency of AI models. AI models require significant computational resources, and the presence of extreme values can exacerbate this, making the models more resource-intensive to run.
By potentially reducing these outliers, the softmax1 function could make AI models more efficient. This would make it more feasible to run complex AI models on devices with limited computational resources, such as smartphones or smaller IoT devices. This could democratize access to AI technology, allowing it to be used in a broader range of applications and settings.
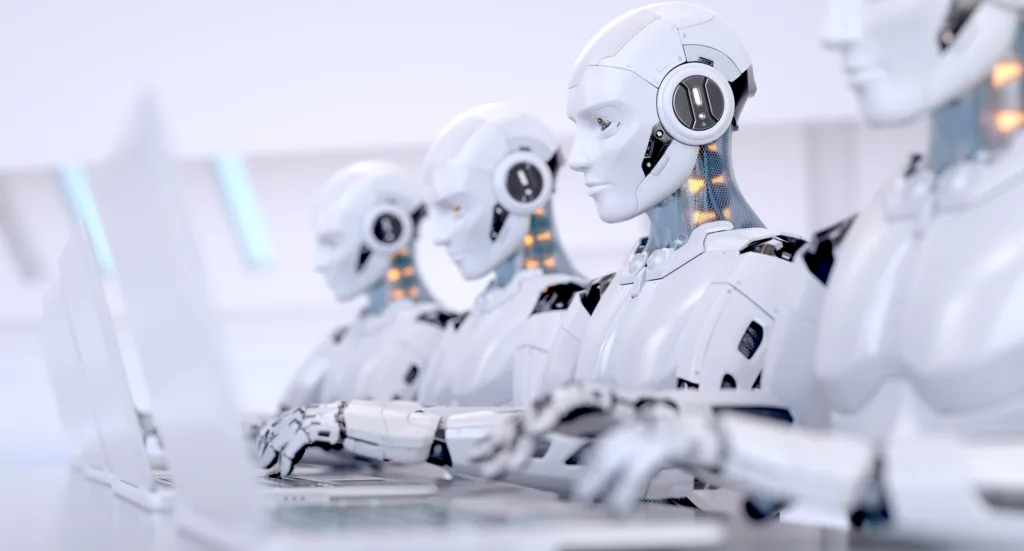
Miller’s findings are a reminder of the importance of continual questioning and innovation in the field of AI. Even seemingly minor tweaks can have significant implications for the functionality, efficiency, and accessibility of AI technology. As AI continues to evolve and become an even more integral part of our lives, it’s crucial that we continue to find new ways to optimize these systems.
Calling All AI Researchers
Miller is encouraging AI researchers to test this new function in their AI models, and he’s optimistic about the results. He suggests that it might improve the performance of these models, making them easier to deploy in various settings, from high-end servers to smaller devices like Raspberry Pis.
Evan Miller’s “Attention Is Off By One” has sparked a new conversation in the AI community. If his claim holds up under further testing, his minor modification could lead to major advancements in AI technology. As with all scientific discoveries, it will require rigorous testing and replication before it can be widely adopted. But if it proves to be as effective as Miller suggests, it could be a game-changer in the world of artificial intelligence.